Pandas Dataframe: Plot Examples with Matplotlib and Pyplot
Last updated: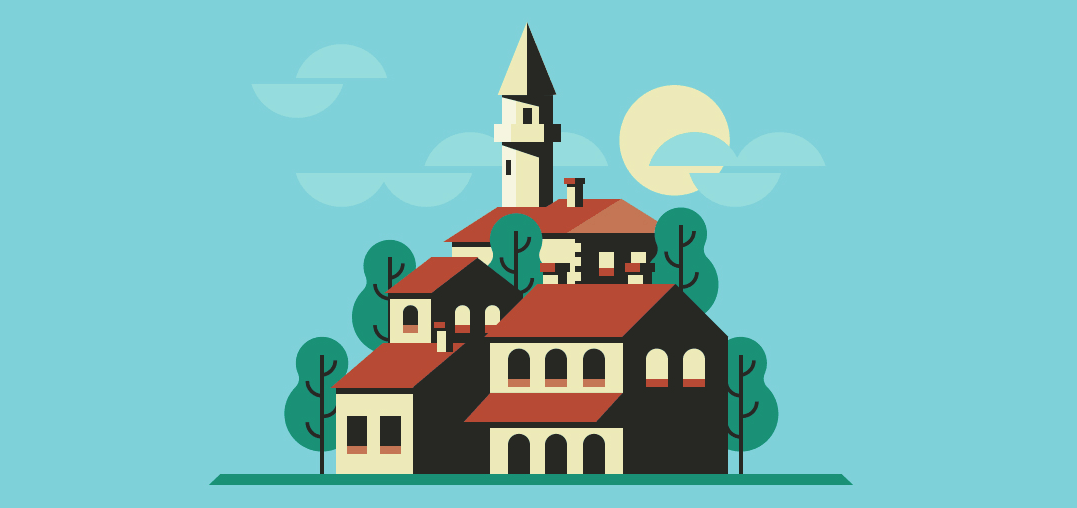
- Scatter plot of two columns
- Bar plot of column values
- Line plot, multiple columns
- Save plot to file
- Bar plot with group by
- Stacked bar plot with group by
- Stacked bar plot with group by, normalized to 100%
- Stacked bar plot, two-level group by
- Stacked bar plot with two-level group by, normalized to 100%
All examples can be viewed in this sample Jupyter notebook
You need to have the matplotlib module installed for this!
Versions used: Pandas 1.x, matplotlib 3.0.x
Sample data for examples
import pandas as pd
df = pd.DataFrame({
'name':['john','mary','peter','jeff','bill','lisa','jose'],
'age':[23,78,22,19,45,33,20],
'gender':['M','F','M','M','M','F','M'],
'state':['california','dc','california','dc','california','texas','texas'],
'num_children':[2,0,0,3,2,1,4],
'num_pets':[5,1,0,5,2,2,3]
})
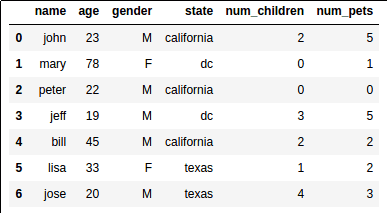
Pandas has tight integration with matplotlib.
You can plot data directly from your DataFrame using the plot()
method:
Scatter plot of two columns
import matplotlib.pyplot as plt
import pandas as pd
# a scatter plot comparing num_children and num_pets
df.plot(kind='scatter',x='num_children',y='num_pets',color='red')
plt.show()
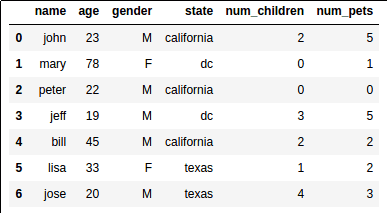
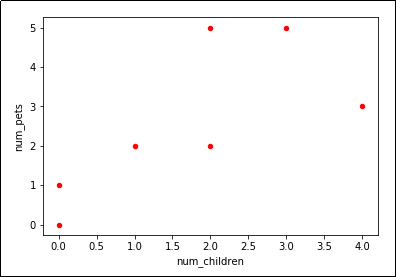
Bar plot of column values
import matplotlib.pyplot as plt
import pandas as pd
# a simple line plot
df.plot(kind='bar',x='name',y='age')
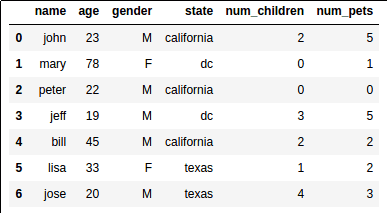
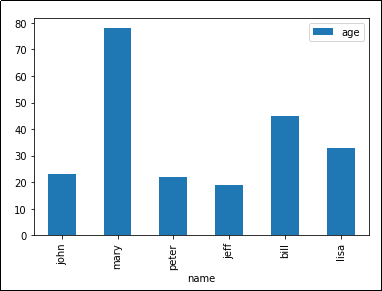
Line plot, multiple columns
Just reuse the Axes
object.
import matplotlib.pyplot as plt
import pandas as pd
# gca stands for 'get current axis'
ax = plt.gca()
df.plot(kind='line',x='name',y='num_children',ax=ax)
df.plot(kind='line',x='name',y='num_pets', color='red', ax=ax)
plt.show()
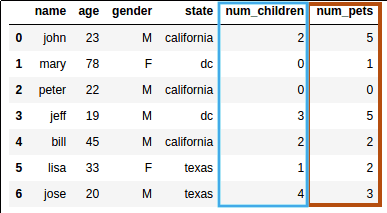
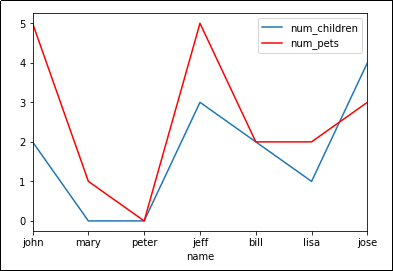
plot()
takes an optional argument 'ax' which allows you to reuse an Axis to plot multiple lines
Save plot to file
Instead of calling plt.show()
, call plt.savefig('outputfile.png')
:
import matplotlib.pyplot as plt
import pandas as pd
df.plot(kind='bar',x='name',y='age')
# the plot gets saved to 'output.png'
plt.savefig('output.png')
Bar plot with group by
import matplotlib.pyplot as plt
import pandas as pd
df.groupby('state')['name'].nunique().plot(kind='bar')
plt.show()
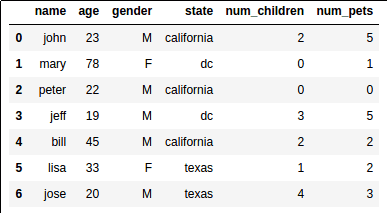
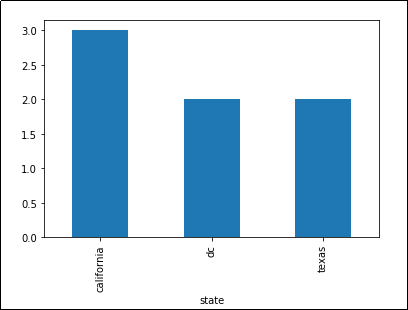
Stacked bar plot with group by
Example: plot count by category as a stacked column:
create a dummy variable and do a two-level group-by based on it:
fix the x axis label and the legend
import matplotlib.pyplot as plt
# create dummy variable them group by that
# set the legend to false because we'll fix it later
df.assign(dummy = 1).groupby(
['dummy','state']
).size().to_frame().unstack().plot(kind='bar',stacked=True,legend=False)
plt.title('Number of records by State')
# other it'll show up as 'dummy'
plt.xlabel('state')
# disable ticks in the x axis
plt.xticks([])
# fix the legend
current_handles, _ = plt.gca().get_legend_handles_labels()
reversed_handles = reversed(current_handles)
labels = reversed(df['state'].unique())
plt.legend(reversed_handles,labels,loc='lower right')
plt.show()
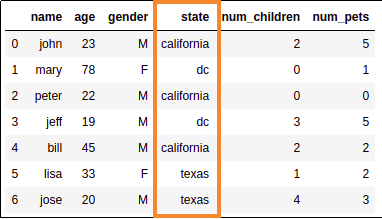
2 for DC and texas
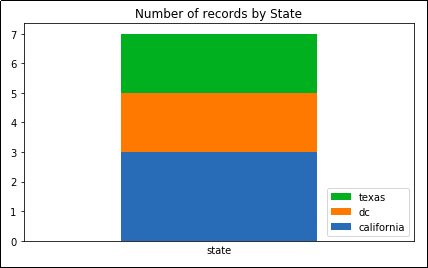
same order as the actual column.
This makes your plot easier to read.
Stacked bar plot with group by, normalized to 100%
A plot where the columns sum up to 100%.
Similar to the example above but:
normalize the values by dividing by the total amounts
use percentage tick labels for the y axis
Example: Plot percentage count of records by state
import matplotlib.pyplot as plt
import matplotlib.ticker as mtick
# create dummy variable then group by that
# set the legend to false because we'll fix it later
df.assign(dummy = 1).groupby(
['dummy','state']
).size().groupby(level=0).apply(
lambda x: 100 * x / x.sum()
).to_frame().unstack().plot(kind='bar',stacked=True,legend=False)
# or it'll show up as 'dummy'
plt.xlabel('state')
# disable ticks in the x axis
plt.xticks([])
# fix the legend or it'll include the dummy variable
current_handles, _ = plt.gca().get_legend_handles_labels()
reversed_handles = reversed(current_handles)
correct_labels = reversed(df['state'].unique())
plt.legend(reversed_handles,correct_labels)
plt.gca().yaxis.set_major_formatter(mtick.PercentFormatter())
plt.show()
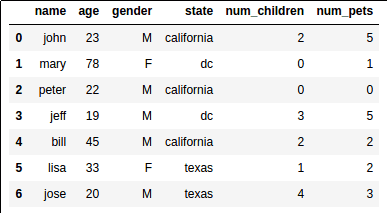
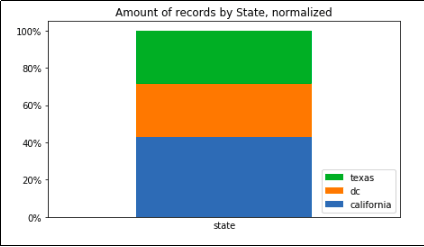
Stacked bar plot, two-level group by
Just do a normal groupby()
and call unstack()
:
import matplotlib.pyplot as plt
import pandas as pd
df.groupby(['state','gender']).size().unstack().plot(kind='bar',stacked=True)
plt.show()
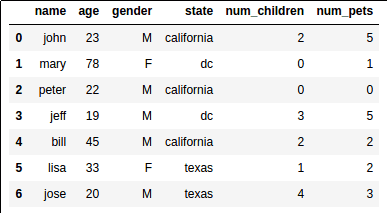
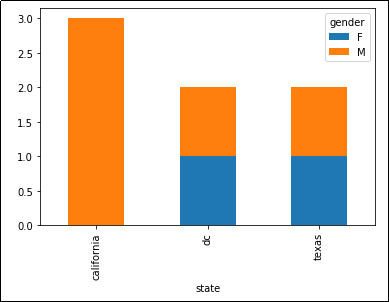
per state, split into males and females
Another example: count the people by gender, spliting by state:
import matplotlib.pyplot as plt
import pandas as pd
df.groupby(['gender','state']).size().unstack().plot(kind='bar',stacked=True)
plt.show()
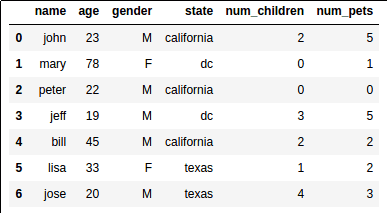
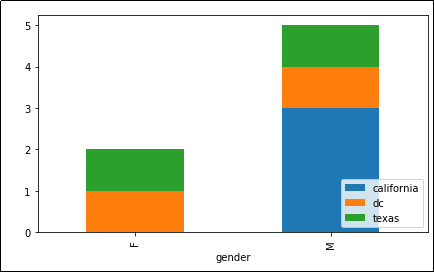
'state'
and 'gender'
Stacked bar plot with two-level group by, normalized to 100%
Sometimes you are only ever interested in the distributions, not raw amounts:
import matplotlib.ticker as mtick
import matplotlib.pyplot as plt
df.groupby(['gender','state']).size().groupby(level=0).apply(
lambda x: 100 * x / x.sum()
).unstack().plot(kind='bar',stacked=True)
plt.gca().yaxis.set_major_formatter(mtick.PercentFormatter())
plt.show()
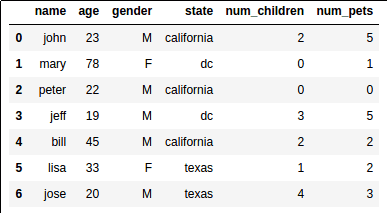
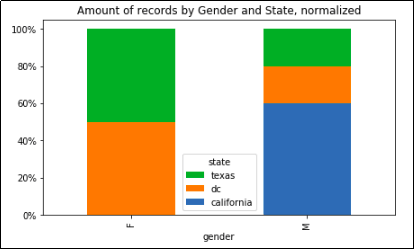
so that each sums up to 100%