Feature Scaling: Quick Introduction and Examples using Scikit-learn
Last updated:WIP Alert This is a work in progress. Current information is correct but more content may be added in the future.
WHY:
Feature scaling helps avoid problems when some features are much larger (in absolute value) than other features.
You need it for all techniques that use distances in any way (i.e. which are scale-variant) such as:
SVM (Support Vector Machines)
k-Nearest Neighbours
PCA (Principal Component Analysis)
You must perform feature scaling in any technique that uses SGD (Stochastic Gradient Descent), such as:
- Neural networks
- Logistic Regresssion
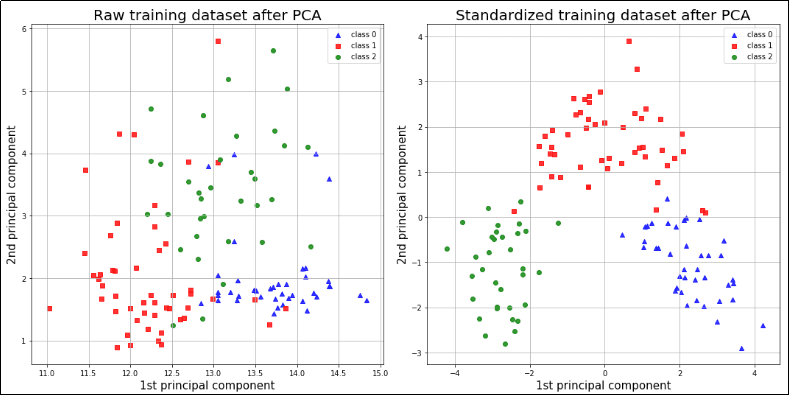
they were applied on standardized or raw features. *
Remember to scale train/test data separately, otherwise you're leaking data!
Simple Feature Recaling
Also called min-max Scaling
WHAT:
Subtract the minimum value and divide by the total feature range (max-min).
Transformed features now lie between 0 and 1
WHEN TO USE:
- TODO
NOTES:
- Heavily influenced by outliers.
EXAMPLE:
from sklearn.preprocessing import MinMaxScaler
scaler = MinMaxScaler()
scaler.fit(X_train)
X_train = scaler.transform(X_train)
X_test = scaler.transform(X_test)
Standardization
WHAT:
- Subtract the mean and divide by the standard deviation.
WHEN TO USE:
When your data has many outliers.
When you need your data to have zero mean.
EXAMPLE:
from sklearn.preprocessing import StandardScaler
scaler = StandardScaler()
scaler.fit(X_train)
X_train = scaler.transform(X_train)
X_test = scaler.transform(X_test)
Normalization
WHAT:
Scale every feature vector so that it has norm = 1.
Usually you'll use L2 (euclidean) norm but you can also use others.
WHEN TO USE:
- When you are going to apply methods such as dot products on the feature vectors.
NOTES:
- Because this transformation does not depend on other points in your dataset, calling
.fit()
has no effect.
EXAMPLE:
from sklearn.preprocessing import Normalizer
normalizer = Normalizer()
# this does nothing because this method doesn't 'train' on your data
normalizer.fit(X_train)
X_train = normalizer.transform(X_train)
X_test = normalizer.transform(X_test)
Robust Scaling
TODO
TODO
CHECK THE VALIDITY OF THIS INFO
source: https://medium.com/towards-data-science/top-6-errors-novice-machine-learning-engineers-make-e82273d394db
READ INTO:
L1/L2 Regularization without standardization
MENTION CONNECTION BETWEEN L1/L2 REGULARIZATION AND NORMALIZATION
L1 and L2 regularization penalizes large coefficients and is a common way to regularize linear or logistic regression; however, many machine learning engineers are not aware that is important to standardize features before applying regularization.