Spark SQL date/time Arithmetic examples: Adding, Subtracting, etc
Last updated:- Summary
- Add/Subtract days to date
- Datediff example
- Datediff versus current timestamp
- Difference in seconds
- Difference in milliseconds
- Difference in hours
- Expr INTERVAL example
Spark 2.4.8 used
See all examples on this jupyter notebook
Summary
Method | Description | |
---|---|---|
date_add(col, num_days) and date_sub(col, num_days) |
Add or subtract a number of days from a date/timestamp. Works on Dates, Timestamps and valid date/time Strings. When used with Timestamps, the time portion is ignored. |
Example |
datediff(col_before, col_after) |
Returns the number of days between two datetime columns. Works on Dates, Timestamps and valid date/time Strings. When used with Timestamps, the time portion is ignored. |
Example |
unix_timestamp(date_col) and subtract |
Returns the number of seconds between two date/time columns. Works on Dates, Timestamps and valid date/time Strings. |
Example |
Cast to double , subtract, multiply by 1000 and cast back to long |
Returns the number of milliseconds between two Timestamp columns. Works on Timestamps only. |
Example |
expr("INTERVAL...") |
Precise subtraction or addition to a Timestamp, without ignoring the time portion. Works on Dates, Timestamps and valid date/time Strings. |
Example |
Add/Subtract days to date
HEADS-UP Time information is ignored. Timestamps are truncated to the day.
Use date_add(source_column, num_days)
or date_sub(source_column, num_days)
Can be used on Date, Timestamp and String columns (when string is a valid date/timestamp string)
import java.sql.Timestamp
import org.apache.spark.sql.functions.date_add
val df = Seq(
("notebook", Timestamp.valueOf("2019-01-29 12:00:00")),
("notebook", Timestamp.valueOf("2019-01-01 00:00:00")),
("small_phone", Timestamp.valueOf("2019-01-15 23:00:00")),
("small_phone", Timestamp.valueOf("2019-01-01 09:00:00"))
).toDF("device", "purchase_time").sort("device","purchase_time")
df.withColumn("plus_2_days",date_add($"purchase_time",2))
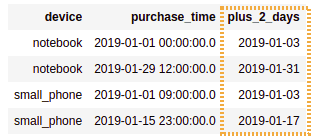
purchase_time
. Time information is lost in the process, and the created column
is always of time
Date
Datediff example
HEADS-UP Time information is ignored. Timestamps are truncated to the day.
Returns the number of days between two dates.
Template: datediff($"column_AFTER", $"column_BEFORE")
import java.sql.Date
import org.apache.spark.sql.functions.datediff
val df = Seq(
("notebook", Date.valueOf("2019-01-29"), Date.valueOf("2019-02-10")),
("notebook", Date.valueOf("2019-01-01"), Date.valueOf("2019-01-15")),
("small_phone", Date.valueOf("2019-01-15"), Date.valueOf("2019-01-05")),
("small_phone", Date.valueOf("2019-01-01"), Date.valueOf("2019-01-20"))
).toDF("device", "purchase_date", "arrival_date").sort("device","purchase_date")
df.withColumn("days_to_arrive",datediff($"arrival_date", $"purchase_date"))
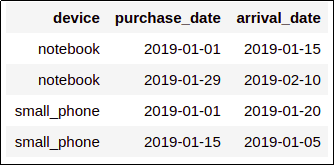
when a product was purchased and
another one with the date it
arrived at the buyer's house.
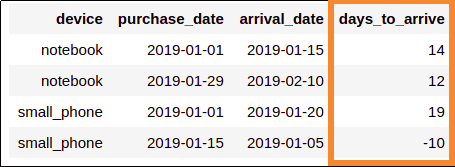
datediff
to calculate the number of days it took
for a package to arrive.
Negative values here probably
indicate a bug somewhere
in the source system code
Datediff versus current timestamp
In other words, number of of days ago. Use datediff
and current_date
.
import java.sql.Date
import org.apache.spark.sql.functions.{datediff, current_date}
val df = Seq(
("notebook", Date.valueOf("2019-01-29"), Date.valueOf("2019-02-10")),
("notebook", Date.valueOf("2019-01-01"), Date.valueOf("2019-01-15")),
("small_phone", Date.valueOf("2019-01-15"), Date.valueOf("2019-01-05")),
("small_phone", Date.valueOf("2019-01-01"), Date.valueOf("2019-01-20"))
).toDF("device", "purchase_date", "arrival_date").sort("device","purchase_date")
df.withColumn("days_since_arrival",datediff(current_date, $"purchase_date"))
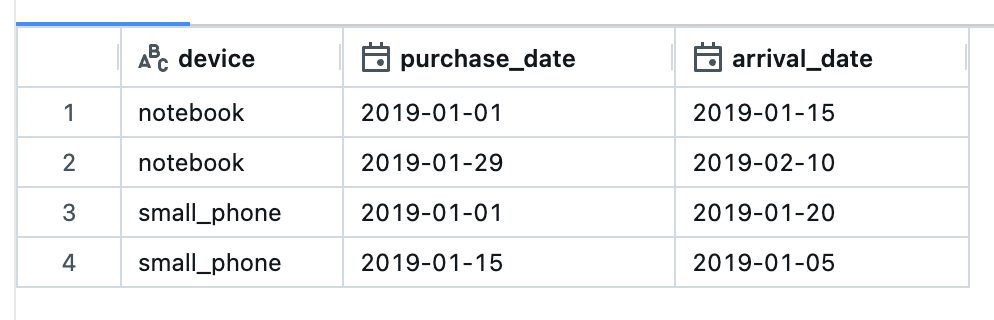
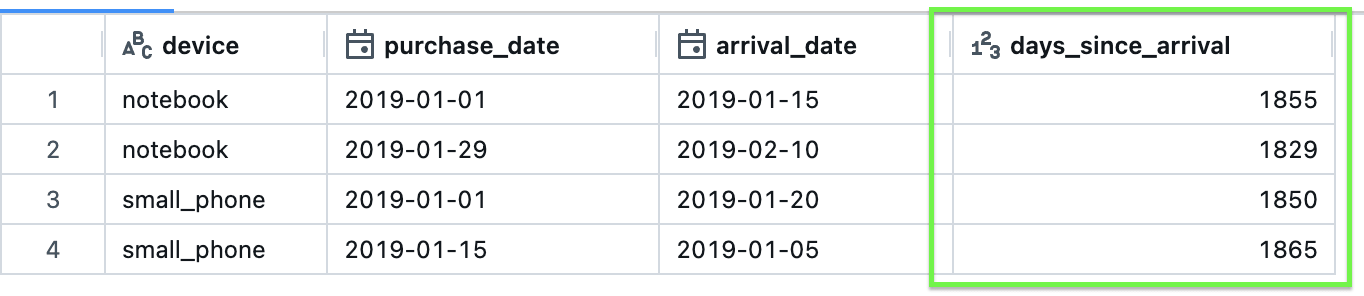
current_timestamp
to get the number of days from the current date
Difference in seconds
- First convert datetime to unix timestamp using
unix_timestamp(datetime_col)
- Then subtract
import java.sql.Timestamp.valueOf
import org.apache.spark.sql.functions.unix_timestamp
val df = Seq(
("foo", valueOf("2019-01-01 00:00:00"), valueOf("2019-01-01 01:00:00")), // 1 hour apart
("bar", valueOf("2019-01-01 00:00:00"), valueOf("2019-01-02 00:00:00")), // 24 hours apart
("baz", valueOf("2019-01-01 00:00:00"), valueOf("2019-01-07 00:00:00")) // 7 days apart
).toDF("col1", "purchase_time", "arrival_time").sort("col1", "purchase_time")
df
.withColumn("diff_in_seconds", unix_timestamp($"arrival_time") - unix_timestamp($"purchase_time"))
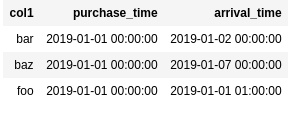
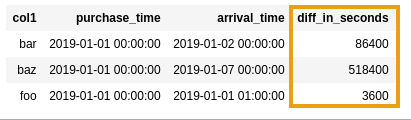
purchase_time
and
arrival_time
Difference in milliseconds
- 1) Cast
Timestamp
todouble
- 2) Subtract both values
- 3) Multiply by 1000
- 4) Cast back to
long
import java.sql.Timestamp.valueOf
import org.apache.spark.sql.functions.to_timestamp
// building the sample dataframe
val df = Seq(
("foo", valueOf("2019-01-01 00:00:00.000"), valueOf("2019-01-01 00:00:00.400")),
("bar", valueOf("2019-01-01 00:00:00.000"), valueOf("2019-01-01 00:00:00.650")),
("baz", valueOf("2019-01-01 00:00:00.000"), valueOf("2019-01-01 00:01:00.000"))
).toDF("col1", "time_before", "time_after")
// cast to double, subtract, multiply by 1000, cast back to long
df
.withColumn("diff_millis", $"time_after".cast("double") - $"time_before".cast("double"))
.withColumn("diff_millis", ($"diff_millis"*1000).cast("long"))
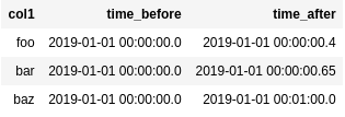
timestamp
columns with microseconds
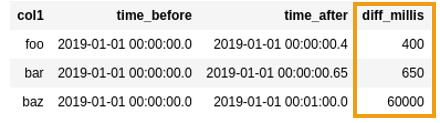
difference between the two timestamp
columns (in milliseconds)
Difference in hours
- Convert to seconds with
cast("double")
- Subtract
- Divide by 36001
import java.sql.Timestamp.valueOf
import org.apache.spark.sql.functions.expr
val df = Seq(
("foo", valueOf("2019-10-10 00:00:00.000"), valueOf("2019-10-10 01:00:00.000")), // exactly 1 hour
("bar", valueOf("2019-10-10 00:00:00.000"), valueOf("2019-10-10 01:00:00.123")), // one hour and some millis
("baz", valueOf("2019-10-10 00:00:00.000"), valueOf("2019-10-11 01:30:00.123")) // one day and one and a half hours
).toDF("col1", "purchase_time", "arrival_time")
val seconds_in_hour = 60*60
df
.withColumn("difference_in_seconds", $"arrival_time".cast("double") - $"purchase_time".cast("double"))
.withColumn("difference_in_hours", $"difference_in_seconds" / seconds_in_hour)
.select("col1", "purchase_time", "arrival_time", "difference_in_hours")
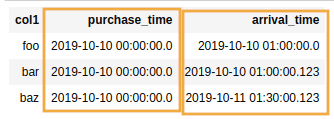
time it arrived at the client
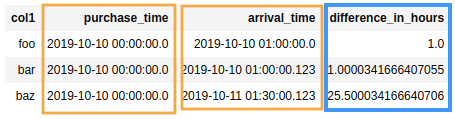
signal when the difference in times is not
a full hour.
Expr INTERVAL example
If you use date_sub
(or date_add
) on timestamps, they get truncated to the date at zero hours.
To avoid truncation (i.e. to subtract a precise period) use $"colname" - expr("INTERVAL ...")
.
Example: subtract 24 hours from timestamp:
import java.sql.Timestamp
import org.apache.spark.sql.functions.expr
val df = Seq(
("foo", Timestamp.valueOf("2019-10-10 00:45:00")),
("bar", Timestamp.valueOf("2019-10-10 12:34:56")),
("baz", Timestamp.valueOf("2019-10-10 23:59:00"))
).toDF("col1", "timestamp_col")
// naturally you can also use "+" for adding periods
// instead of subtracting
df
.withColumn("timestamp_minus_24_hours", $"timestamp_col" - expr("INTERVAL 24 HOURS"))
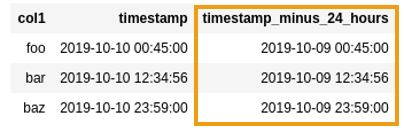
use
$"col" - expr("INTERVAL 24 HOURS")
1: 3600 (60*60) is the number of seconds in an hour.