Michelangelo Palette Overview
Last updated:- Stages of the ML model lifecyle
- Palette
- The data stores
- Types of features
- Custom features
- Feature Post-processing
- Takeaways
This post is based off a 2019 talk by Uber, available online at InfoQ: Michelangelo Palette: A Feature Engineering Platform at Uber
Stages of the ML model lifecyle
Source: Slides from Uber presentation here
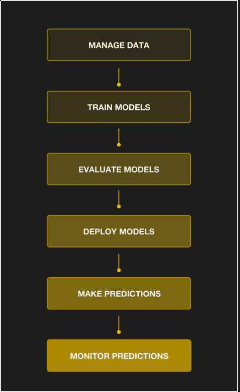
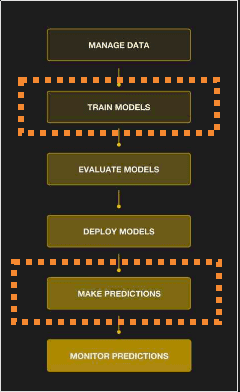
raw data when training models but
also when serving predictions
Palette
Palette is Michelangelo's Feature Store.
Centralized: Single source of truth for features; can be used by all teams across Uber. Less rework, more consistency, etc.
Catalogs: Features are grouped into perspectives, e.g. features for riders, for trips, for drivers, etc.
Training/Serving Skew: Features used at training time are the same as those used at serving time.
The data stores
Feature data is stored in a Cassandra database.
Features are identified according to an expression: @palette:<catalog>:<group>:<feature_name>:<join_key>
For example @palette:restaurant:realtime_group:orders_last_30_min:restaurant_uuid
which means: a feature that stores the number of orders in the last 30 minutes for a given restaurant, uniquely identified by a restaurant_uuid
1
Types of features
Uber splits features into two types:
Batch features are not sensitive to time, i.e. features that don't vary with time. You'll get the same result no matter at what time you ask for it.
- Built on Hive and/or Spark
- Examples: Size of a given restaurant, Average number of orders per week in a given restaurant, average number of rides per day for a given driver
Real-time Features vary depending on the time. Need to be computed on demand.
- Built on Flink streams
- Examples: Number of rides in the last hour for a given driver, average number of meals
Custom features
Palette supports custom features, i.e. you can have Palette call an API you control to fetch a custom feature not available in the main feature store.
Users (i.e. Uber engineers/scientists using Michelangelo) are responsible for keeping the quality of these custom features.
Feature Post-processing
Sometimes features are not ready for consumption (training/serving), they need to be further processed.
Palette has so-called consumption pipelines3 which enable users to define extra feature processing steps.
These are also represented in the same way as features, and are mapped to a Spark UDF in the backend.
Examples:
Missing value imputation
- Mean, Mode, Median, etc.
Joined features
- For example, you have an order id but you want to retrieve features for the restaurant that shipped that meal.
Function calls
- For example, you have a restaurant id but the feature you want to use is the average busyness2 of the region where the restaurant is located. This means that you first need to call a function that returns the region for the restaurant and then fetching the region feature.
One-hot encoding
Takeaways
Offline/online parity is crucial
Optimize speed for real-time features, optimize scale for offline features
Use paralell IO and caching for faster serving of online features
Feature stores make it easier to setup model monitoring (bad data, drift, etc) out of the box
1: This is taken from the presentation slides, but I think they forgot to add a timestamp to the primary key, because the number of orders in the last thirty minutes will naturally be different for the same restaurant if you query it at different timestamps.
2: I.e. how busy a given region is with respect to traffic, number of orders, etc.
3: Mostly Spark ML Pipelines