Mutate for Pandas Dataframes: Examples with Assign
Last updated:Table of Contents
All examples on this jupyter notebook
mutate
is a very popular function is R's dplyr package.
Since many people are familiar with R and would like to have similar behaviour in pandas (it's also useful for those who've never used R).
Pandas assign() function is the equivalent of mutate
for pandas.
Why use assign?
You can use it to avoid needing to define tons of intermediate dataframes in your code, especially if you're using Jupyter Notebooks or similar exploratory tools.
Assign example
Template:
df.assign(column_name = function_that_takes_a_dataframe_and_returns_a_series)
import pandas as pd
df = pd.DataFrame({
'name': ['alice','bob','charlie','daniel'],
'age': [25,66,56,78]
})
df.assign(
is_senior = lambda dataframe: dataframe['age'].map(lambda age: True if age >= 65 else False)
)
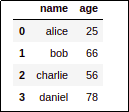
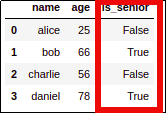
using the assign method
Chain application
import pandas as pd
df = pd.DataFrame({
'name': ['alice','bob','charlie','daniel'],
'age': [25,66,56,78]
})
df.assign(
is_senior = lambda dataframe: dataframe['age'].map(lambda age: True if age >= 65 else False)
).assign(
name_uppercase = lambda dataframe: dataframe['name'].map(lambda name: name.upper()),
).assign(
name_uppercase_double = lambda dataframe: dataframe['name_uppercase'].map(lambda name: name.upper()+"-"+name.upper())
)
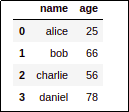
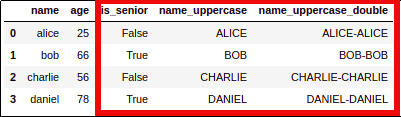
.assign
you can make multiple operations that depend on the
previous ones without the need
of creating intermediate variables