Paper Summary: Cross-Task Generalization via Natural Language Crowdsourcing Instructions
Last updated:Please note This post is mainly intended for my personal use. It is not peer-reviewed work and should not be taken as such.
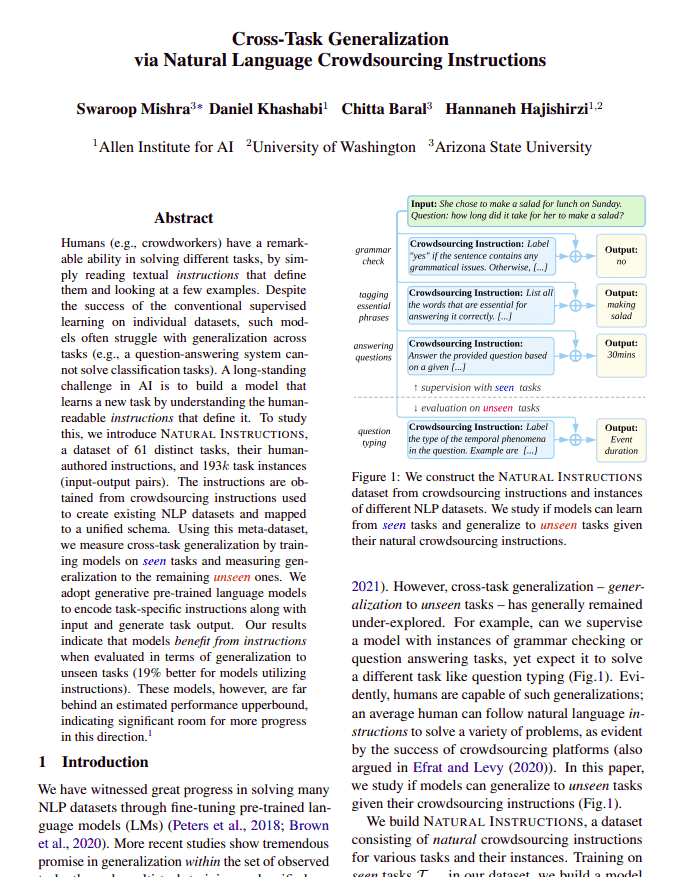
WHAT
Build a dataset with pairs of high-quality instruction-following examples;
Measure how fine-tuned models perform when trained to follow those instructions.
WHY
To provide a dataset for other people to build up on.
To examine the tradeoff between fine-tuning a smaller model vs using a much larger model
HOW
Build a dataset with examples of instructions and fine-tune a pre-trained LM on those
The datasets consist of instructions and task examples, so models are queried in a few-shot setting.
CLAIMS
LMs fine-tuned for instruction-following can generalize into task instances and even task types not seen in the training dataset.
A 170M-parameter model (BART), when fine-tuned, is better at following instructions than GPT-3 with 175B parameters.
EXTENDS/USES
- BART LM(Lewis et al., 2019)
QUOTES
- Authors didn't try to fine-tune GPT-3, apparently because they didn't have enough compute resources "We cannot fine-tune the parameters of [GPT-3] and use it as-is under its default setting"
NOTES
Uses ROUGE for evaluation (generated vs actual)
Examples in the evaluation set are not from different tasks as those in the training set—they are different examples of the same tasks.
MY 2¢
Why don't people use this preference dataset more often?
This is an updated version of a 2021 paper called "Natural Instructions: Benchmarking generalization to new tasks from natural language instructions". It is sometimes referenced by its old name.