Paper Summary: Zephyr: Direct Distillation of LM Alignment
Last updated:Please note This post is mainly intended for my personal use. It is not peer-reviewed work and should not be taken as such.
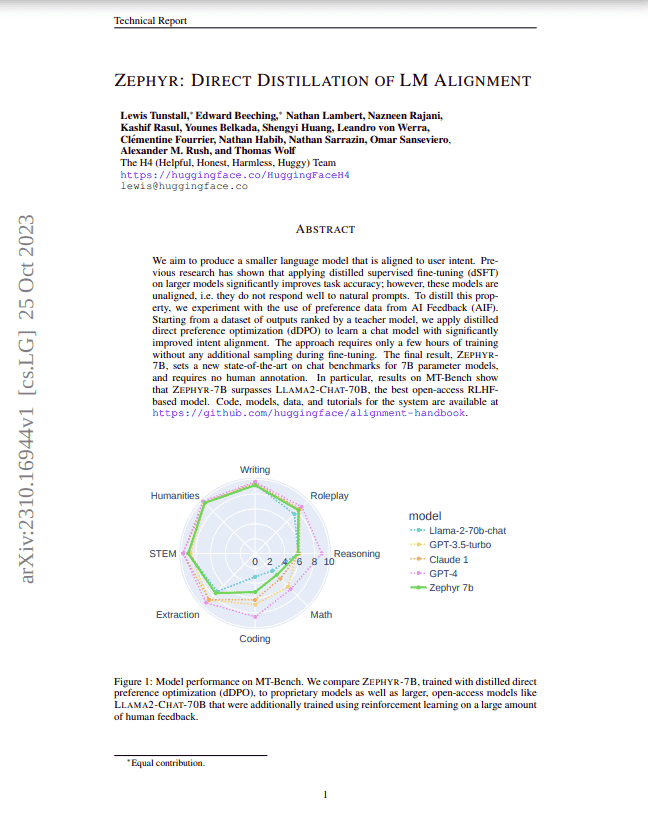
WHAT
Authors instruction-tune Mistral-7B vanilla by distillation: using DPO on open preference datasets and samples generated from previously aligned teacher models.
WHY
Because traditional distillation strategies are only good at transferring stylistic — not alignment capabilities.
HOW
Starting with Mistral-7B as the V0 model:
1) Run SFT on V0 using input/output pairs from the UltraChat dataset, generating model V1
3) Use inputs from UltraFeedback dataset and, for each input, feed it to intermediary models (Claude, Falcon, etc), generating multiple output variations for the same input.
4) For each input from step 3, feed all the output variations to the teacher model (GPT-4) and ask it to select the best one.
5) Use DPO to align model V1, using the best output for each input, as selected on step 4.1
CLAIMS
It's possible to transfer alignment capabilities from teacher models using the suggested approach.
The DPO model overfits quickly with longer training.
Zephyr-7B outperforms 70B models (such as Llama-chat-70B) on some benchmarks.
QUOTES
- "... without an initial SFT step ... models are not able to learn at all from feedback and perform terribly."
- This is interesting. We can't jump to reward modeling without the initial SFT step.
EXTENDS/USES
Mistral-7B
Other aligned LLMs as teachers: Claude, Falcon, Llama, GPT-4.
NOTES
Distillation appears to be the default term for extracting the capabilities of a "teacher" model into a simpler and cheaper "student" model. Apparently it was introduced by Hinton et al 2015.
Zephyr-7B was fully optimized for Helpfulness only.
1: More precisely, DPO is optimized using the best response to each each, but contrasting it to a randomly chosen response. It doesn't classify response, it ranks them